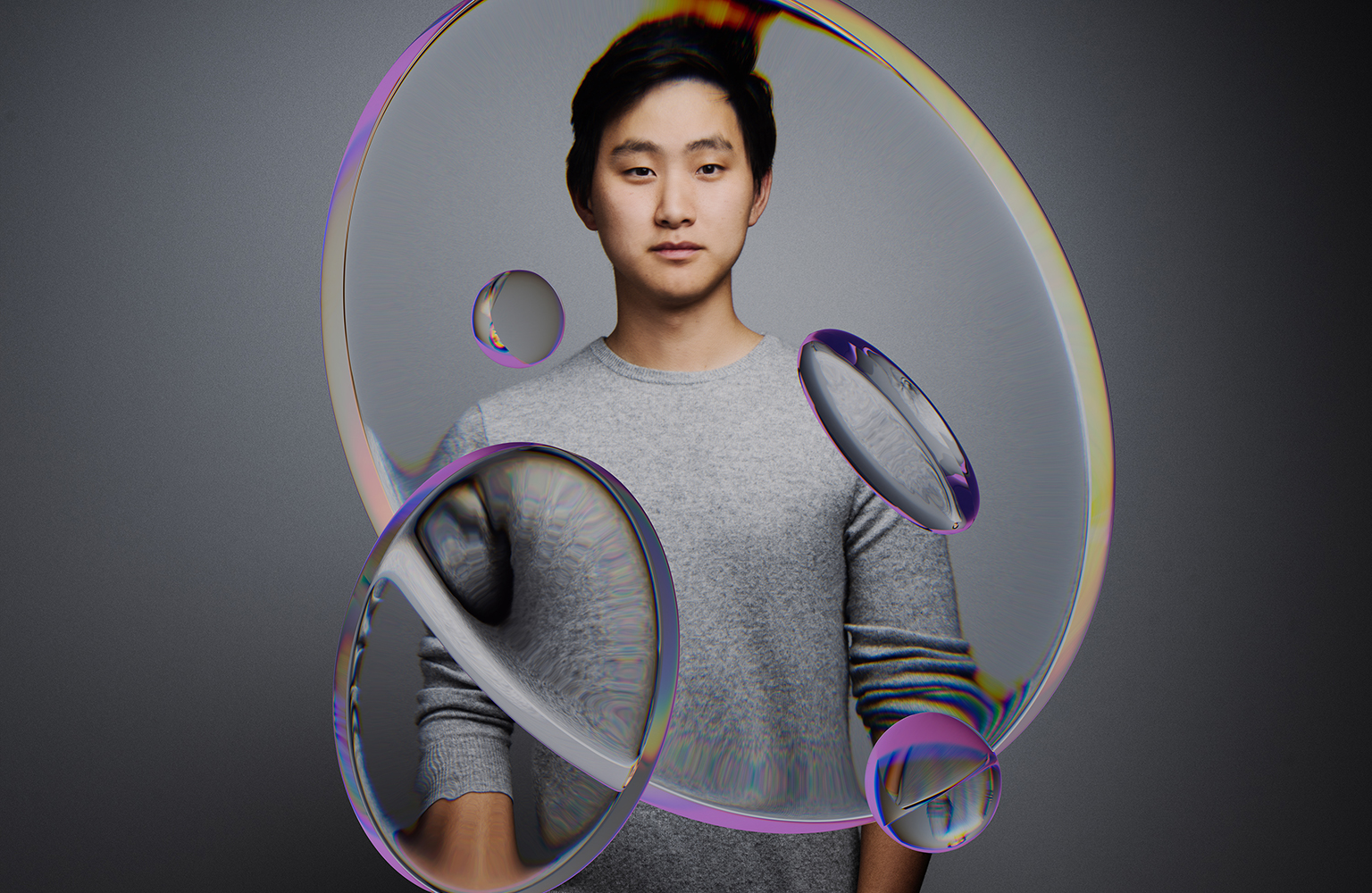
Our Investment in Scale AI
Data is a key pillar for performance in machine learning and generative AI (GenAI) models, but making the vast troves of data usable by these models is a non-trivial task. As GenAI models continue to explode in size, the data needed to train, evaluate, and fine-tune these models for specific use cases will also grow exponentially. This need will be exacerbated by the nature of images, videos, audio, and other complex multi-modal datasets.
At Intel Capital, we believe that we have only utilized the tip of the iceberg in terms of leveraging high-quality data in GenAI models to date; with the increased availability of proprietary, synthetic, and other forms of frontier data, enterprises will need a platform to fully leverage these datasets across the AI lifecycle. That’s why we’re thrilled to announce our Series F investment in Scale AI.
Traditional machine learning utilizes narrow and specific models that are trained primarily on structured data sets. While the quality of these models is driven by the data they are trained on, their performance plateaus at a certain level of data. As such, where data is the new oil, these models are like go-karts that can only go 30 mph around a track.
Foundation models (FMs) are different. FMs, most of which are built leveraging the transformer architecture, are general purpose models that are trained on large corpuses of data. Notably, their performance scales immensely with the amount of data they are trained on, with FMs exhibiting emergent behaviors that researchers did not expect from these models. Accordingly, using the data as the oil metaphor, FMs are like gas guzzlers that aren’t satiated by the oil they are fed, and can surprisingly take off into outer space with the right amount and type of oil. This emergent behavior has resulted in a race to get as much data as possible to feed these FMs.
Some researchers believe that the industry’s need for data will outstrip the supply of data in the coming years. OpenAI’s GPT-4 was trained on as many as 12 trillion tokens, and it is estimated that GPT-5 would need 60 trillion to 100 trillion tokens of data. Synthetic data and proprietary data providers have emerged to fill this shortfall. However, this data needs to be curated, annotated, and contextualized. Further, GenAI models improve iteratively using feedback from humans or other ML models. Lastly, pre-trained FMs need to be fine-tuned for specific use cases within enterprises, using data and instructions that are proprietary to those enterprises. These novel requirements around data use for GenAI are being met by Scale AI.
Scale AI has played a major role in advancing AI in the last few years - beginning with computer vision for autonomous driving to being a trusted partner for the world’s top GenAI companies, U.S. government agencies, and enterprises.
Scale AI’s product vision established the company as a category leader, and will continue to propel the development of AI models and applications of the future. We are beyond thrilled to be partnering with them on this journey.