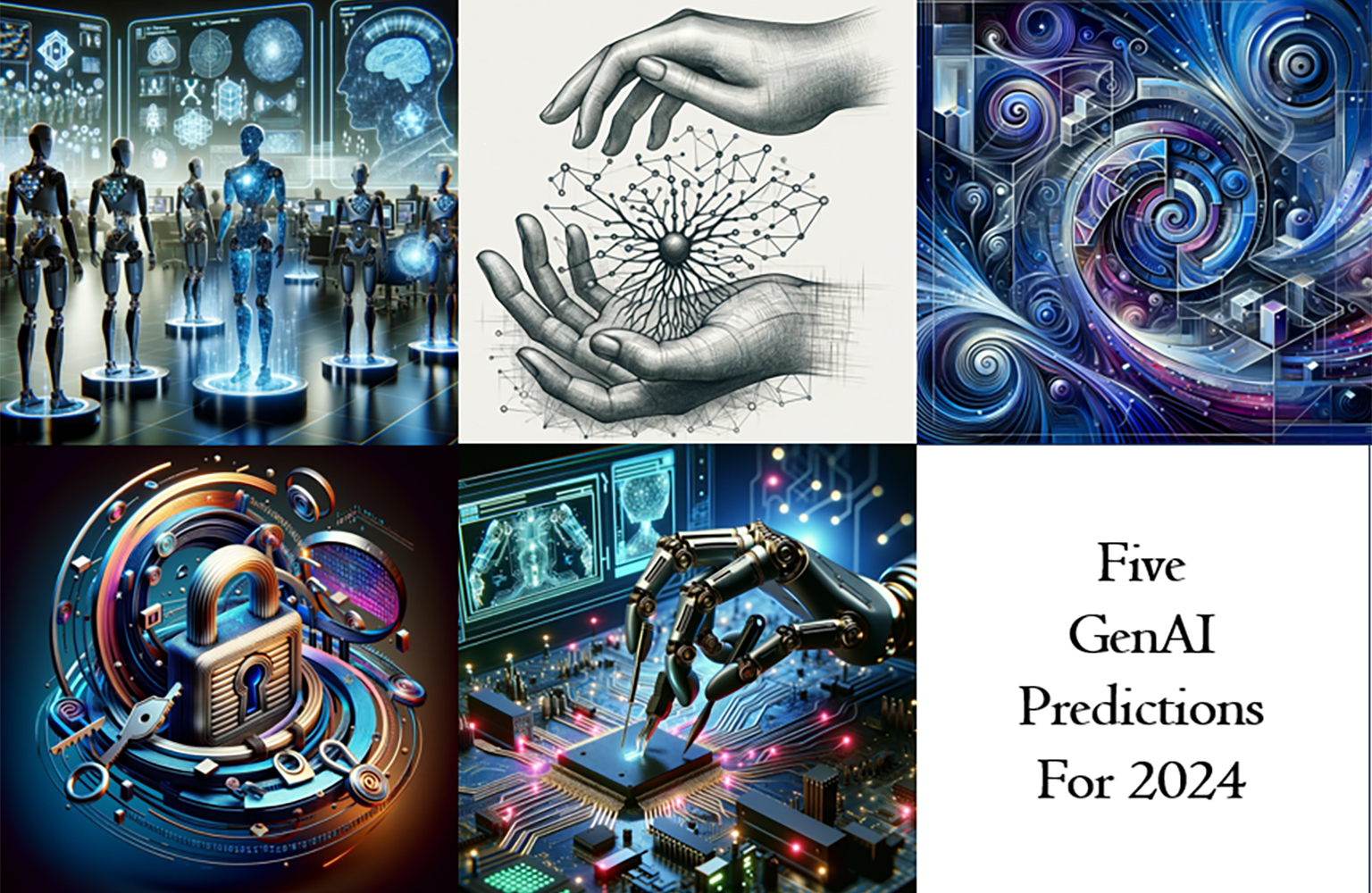
Five GenAI Predictions for 2024
Taking stock of the breakthrough pace of innovation in recent years, here are my five predictions for how generative AI will evolve in 2024.
TL;DR:
1. 🤖 Agentic architectures will mature and see increased adoption
2. 🔦The race to find alternatives to the Transformer will accelerate
3. 🔐 Startups will prioritize copyright, privacy and access control issues to unlock enterprise adoption
4. 🔄Workflows in inefficient domains will be rewritten
5. 🗜 Enterprises will shift to small models (SLMs) for many use cases
Last year's GenAI narrative was one of breathtaking innovation and experimentation, where people had little time to pause and take stock of the best ways to incorporate GenAI in their domains. This year, however, promises a phase of consolidation and reflection, as users across various sectors aim to more deliberately integrate and harness the power of GenAI, optimizing its benefits while navigating its complexities. Here are my five predictions for how GenAI will evolve in 2024.
1. 🤖 Agentic architectures will mature and see increased adoption
Agent-based architectures are poised for significant maturation, as the advantages of specialized, narrowly defined agents over general-purpose systems become increasingly apparent. These specialized agents, chained together, can tackle complex tasks with efficiency and precision unattainable by singular, broad-scope systems. This evolution will manifest in both the infrastructure and application layers. At the infrastructure level, advancements will address critical challenges such as memory and state management, error handling, and system evaluations, ensuring the architecture's reliability, efficiency, and scalability. These improvements will lay a robust foundation that supports complex inter-agent operations seamlessly. Some companies building at the forefront of this space include SuperAGI, Faktory, Sema4.ai, and Plastic Labs. At the application layer, leveraging agent-based architectures will unlock new potentials for developing solutions that require high degrees of customization and adaptability. This approach allows for the creation of applications capable of performing highly complex and precise tasks autonomously.
2. 🔦The race to find alternatives to the Transformer will accelerate
The pace at which alternatives to the transformer architecture are being developed is set to increase rapidly. The transformer model, foundational to nearly all current generative AI tools, excels in processing sequential data in parallel, a feature that enables it to manage long-range dependencies and understand the positional context within sequences effectively. However, this strength also poses a significant limitation, as the transformer's efficiency diminishes at scale. Recent months have seen a surge in research aimed at uncovering viable alternatives to the transformer model, with several projects demonstrating capabilities that surpass the traditional transformer in terms of scalability, throughput, and latency. Among the notable teams contributing to this space are Cartesia, Together AI, Manifest AI, Recursal, and Liquid AI. Each of these teams brings a unique approach to overcoming the challenges inherent in the transformer architecture, potentially doing to fields like synthetic biology and quantitative finance what ChatGPT did to text.
3. 🔐 Startups will prioritize copyright, privacy and access control issues to unlock enterprise adoption
Enterprises have shown reluctance in fully integrating GenAI technologies, largely due to a range of secondary concerns. For instance, the issue of copyright infringement, both in training and during inference, has come to the forefront through a number of lawsuits filed by content owners in the past few months. Additionally, the potential leakage of proprietary data poses a significant threat, especially when sensitive information is fed into AI models for training or analysis. Executives are also wary of unfair and discriminatory behavior stemming from LLMs, which can reflect or amplify biases present in their training data. These concerns had taken a backseat given the pace of innovation in the field, but that is changing as buyers reprioritize. Startups that hadn’t focused on these issues will need to do so now in order to unlock enterprise adoption. Stay tuned for announcements on this front.
4. 🔄Workflows in inefficient domains will be rewritten
Most industries have rent-seekers who thrive in structurally inefficient workflows. Government contracting/bidding processes, preauthorization of medical procedures, and documentation involved in real estate transactions are just a few examples. There are tens of examples of such practices in industries such as healthcare, financial services, real estate, regulatory compliance, and more. Software powered by foundation models (FMs) can simplify these inefficient workflows and level the playing field. While much of the early focus of FMs was on broad horizontal applications, 2024 will see many startups focusing on these narrower wedges. Some companies innovating in this space are Nabla, Procurement Sciences AI, Sixfold and MaxHome.
5. 🗜 Enterprises will shift to small models (SLMs) for many use cases
Enterprises adopting large FMs are recognizing that these general-purpose FMs come with prohibitive costs. They are finding that for certain use cases, particularly where speed and cost are critical, similar performance can be achieved through smaller models. Additionally, scenarios requiring deployment on smaller edge devices necessitate the use of these SLMs due to hardware constraints. The introduction of techniques such as transfer learning and the development of mixture-of-experts models have significantly enhanced the capabilities of smaller models. These advancements enable them to match, and in many cases, perform as well as their larger counterparts across various applications. More enterprises will embrace SLMs going forward.
If you’re building in any of these (or other) areas of generative AI, my partners and I at Intel Capital would love to chat! You can reach me through LinkedIn or X, formerly known as Twitter.